Please use this identifier to cite or link to this item:
https://hdl.handle.net/11000/30460
Noise-Scaled Euclidean Distance: A Metric for Maximum Likelihood Estimation of the PV Model Parameters
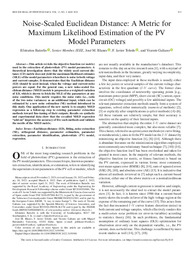
View/Open:
Noise-Scaled_Euclidean_Distance_A_Metric_for_Maximum_Likelihood_Estimation_of_the_PV_Model_Parameters.pdf
1,65 MB
Adobe PDF
Share: