Please use this identifier to cite or link to this item:
https://hdl.handle.net/11000/34275
Full metadata record
DC Field | Value | Language |
---|---|---|
dc.contributor.author | Ballesta, Mónica | - |
dc.contributor.author | Payá, Luis | - |
dc.contributor.author | Cebollada, Sergio | - |
dc.contributor.author | Reinoso, Oscar | - |
dc.contributor.author | Murcia, Francisco | - |
dc.contributor.other | Departamentos de la UMH::Ingeniería de Sistemas y Automática | es_ES |
dc.date.accessioned | 2025-01-10T16:39:03Z | - |
dc.date.available | 2025-01-10T16:39:03Z | - |
dc.date.created | 2021-08 | - |
dc.identifier.citation | Appl. Sci. 2021, 11 | es_ES |
dc.identifier.issn | 2076-3417 | - |
dc.identifier.uri | https://hdl.handle.net/11000/34275 | - |
dc.description.abstract | Understanding the environment is an essential ability for robots to be autonomous. In this sense, Convolutional Neural Networks (CNNs) can provide holistic descriptors of a scene. These descriptors have proved to be robust in dynamic environments. The aim of this paper is to perform hierarchical localization of a mobile robot in an indoor environment by means of a CNN. Omnidirectional images are used as the input of the CNN. Experiments include a classification study in which the CNNis trained so that the robot is able to find out the room where it is located. Additionally, a transfer learning technique transforms the original CNN into a regression CNN which is able to estimate the coordinates of the position of the robot in a specific room. Regarding classification, the room retrieval task is performed with considerable success. As for the regression stage, when it is performed along with an approach based on splitting rooms, it also provides relatively accurate results. | es_ES |
dc.format | application/pdf | es_ES |
dc.format.extent | 17 | es_ES |
dc.language.iso | eng | es_ES |
dc.publisher | MDPI | es_ES |
dc.rights | info:eu-repo/semantics/openAccess | es_ES |
dc.rights.uri | http://creativecommons.org/licenses/by-nc-nd/4.0/ | * |
dc.subject | CNNs | es_ES |
dc.subject | classification | es_ES |
dc.subject | localization | es_ES |
dc.subject | mobile robots | es_ES |
dc.subject | omnidirectional images | es_ES |
dc.subject | transfer learning | es_ES |
dc.subject | regression | es_ES |
dc.subject.other | CDU::6 - Ciencias aplicadas::62 - Ingeniería. Tecnología | es_ES |
dc.title | ACNNRegression Approach to Mobile Robot Localization Using Omnidirectional Images | es_ES |
dc.type | info:eu-repo/semantics/article | es_ES |
dc.relation.publisherversion | https://doi.org/ 10.3390/app11167521 | es_ES |
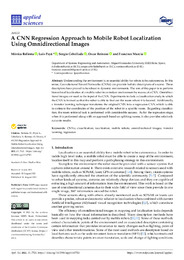
View/Open:
applsci-11-07521-v3 (1) (1).pdf
2,67 MB
Adobe PDF
Share: