Please use this identifier to cite or link to this item:
https://hdl.handle.net/11000/30568
Full metadata record
DC Field | Value | Language |
---|---|---|
dc.contributor.author | Melgarejo Meseguer, Francisco Manuel | - |
dc.contributor.author | Bleda, Andres L. | - |
dc.contributor.author | Abbenante, Sergio | - |
dc.contributor.author | Gimeno Blanes, Francisco Javier | - |
dc.contributor.author | EVERSS, ESTRELLA | - |
dc.contributor.author | Muñoz-Romero, Sergio | - |
dc.contributor.author | Rojo-Álvarez, José Luis | - |
dc.contributor.author | Maestre, Rafael | - |
dc.contributor.other | Departamentos de la UMH::Ingeniería de Comunicaciones | es_ES |
dc.date.accessioned | 2024-01-23T11:37:42Z | - |
dc.date.available | 2024-01-23T11:37:42Z | - |
dc.date.created | 2022-04 | - |
dc.identifier.citation | IEEE Internet of Things Journal VOL. 14, NO. 8, APRIL (2022) | es_ES |
dc.identifier.issn | 2327-4662 | - |
dc.identifier.uri | https://hdl.handle.net/11000/30568 | - |
dc.description.abstract | Human Activity Recognition poses a significant challenge within Active and Assisted Living (AAL) systems, relying extensively on ubiquitous environmental sensor-based acquisition devices to detect user situations in their daily living. Environmental measurement systems deployed indoors yield multiparametric data in heterogeneous formats, which presents a challenge for developing Machine Learning-based AAL models. We hypothesized that anomaly detection algorithms could be effectively employed to create data-driven models for monitoring home environments and that the complex multiparametric indoor measurements can often be represented by a relatively small number of latent variables generated through Manifold Learning (MnL) techniques. We examined both linear (Principal Component Analysis) and non-linear (AutoEncoders) techniques for generating these latent spaces and the utility of core domain detection techniques for identifying anomalies within the resulting low-dimensional manifolds. We benchmarked this approach using three publicly available datasets (hh105, Aruba, and Tulum) and one proprietary dataset (Elioth) for home environmental monitoring. Our results demonstrated the following key findings: (a) Nonlinear manifold estimation techniques offer significant advantages in retrieving latent variables when compared to linear techniques; (b) The quality of the reconstruction of the original multidimensional recordings serves as an acceptable indicator of the quality of the generated latent spaces; (c) Domain detection identifies regions of normality consistent with typical individual activities in these spaces; And (d) the system effectively detects deviations from typical activity patterns and labels anomalies. This study lays the groundwork for further exploration of enhanced methods for extracting information from MnL data models and their application within the AAL and possibly other sectors. | es_ES |
dc.format | application/pdf | es_ES |
dc.format.extent | 12 | es_ES |
dc.language.iso | eng | es_ES |
dc.publisher | Institute of Electrical and Electronics Engineers | es_ES |
dc.rights | info:eu-repo/semantics/openAccess | es_ES |
dc.rights.uri | http://creativecommons.org/licenses/by-nc-nd/4.0/ | * |
dc.subject | Autoencoders | es_ES |
dc.subject | Deep Learning | es_ES |
dc.subject | Human Activity Recognition | es_ES |
dc.subject | Anomaly Detection | es_ES |
dc.subject | Sensors | es_ES |
dc.subject | Active and Assisted Living | es_ES |
dc.subject | Latent Spaces | es_ES |
dc.subject.other | CDU::6 - Ciencias aplicadas::62 - Ingeniería. Tecnología | es_ES |
dc.title | Anomaly Detection from Low-dimensional Latent Manifolds with Home Environmental Sensors | es_ES |
dc.type | info:eu-repo/semantics/article | es_ES |
dc.relation.publisherversion | https://doi.org/10.1109/JIOT.2023.3338697 | es_ES |
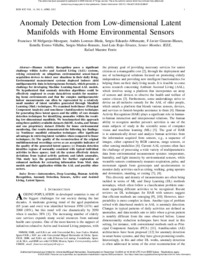
View/Open:
231201 Anomaly_Detection_From_Low-Dimensional_Latent_Manifolds_With_Home_Environmental_Sensors.pdf
5,31 MB
Adobe PDF
Share: