Por favor, use este identificador para citar o enlazar este ítem:
https://hdl.handle.net/11000/35328
Registro completo de metadatos
Campo DC | Valor | Lengua/Idioma |
---|---|---|
dc.contributor.author | Gilly, Katja | - |
dc.contributor.author | Filiposka, Sonja | - |
dc.contributor.author | Alcaraz, Salvador | - |
dc.contributor.other | Departamentos de la UMH::Ingeniería de Computadores | es_ES |
dc.date.accessioned | 2025-01-26T15:42:43Z | - |
dc.date.available | 2025-01-26T15:42:43Z | - |
dc.date.created | 2021 | - |
dc.identifier.citation | Applied Sciences | es_ES |
dc.identifier.issn | 2076-3417 | - |
dc.identifier.uri | https://hdl.handle.net/11000/35328 | - |
dc.description.abstract | Advanced learning algorithms for autonomous driving require lots of processing and storage power, which puts a strain on vehicles’ computing resources. Using a combination of 5G network connectivity with ultra-high bandwidth and low latency together with extra computing power located at the edge of the network can help extend the capabilities of vehicular networks. However, due to the high mobility, it is essential that the offloaded services are migrated so that they are always in close proximity to the requester. Using proactive migration techniques ensures minimum latency for high service quality. However, predicting the next edge server to migrate comes with an error that can have deteriorating effects on the latency. In this paper, we examine the influence of mobility prediction errors on edge service migration performances in terms of latency penalty using a large-scale urban vehicular simulation. Our results show that the average service delay increases almost linearly with the migration prediction error, with 20% error yielding almost double service latency. | es_ES |
dc.format | application/pdf | es_ES |
dc.format.extent | 16 | es_ES |
dc.language.iso | eng | es_ES |
dc.publisher | MDPI | es_ES |
dc.relation.ispartofseries | 11 | es_ES |
dc.relation.ispartofseries | 3 | es_ES |
dc.rights | info:eu-repo/semantics/openAccess | es_ES |
dc.rights | Attribution-NonCommercial-NoDerivatives 4.0 Internacional | * |
dc.rights.uri | http://creativecommons.org/licenses/by-nc-nd/4.0/ | * |
dc.subject | edge computing | es_ES |
dc.subject | migrations | es_ES |
dc.subject | predictive modelling | es_ES |
dc.subject | urban vehicular scenarios | es_ES |
dc.subject.other | CDU::6 - Ciencias aplicadas::62 - Ingeniería. Tecnología | es_ES |
dc.title | Predictive Migration Performance in Vehicular Edge Computing Environments | es_ES |
dc.type | info:eu-repo/semantics/article | es_ES |
dc.relation.publisherversion | https://doi.org/10.3390/app11030944 | es_ES |
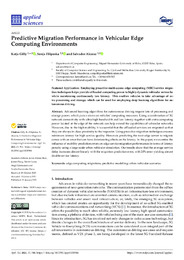
Ver/Abrir:
applsci-11-00944.pdf
1,43 MB
Adobe PDF
Compartir:
La licencia se describe como: Atribución-NonComercial-NoDerivada 4.0 Internacional.