Por favor, use este identificador para citar o enlazar este ítem:
https://hdl.handle.net/11000/35180
Registro completo de metadatos
Campo DC | Valor | Lengua/Idioma |
---|---|---|
dc.contributor.author | Lozano, Antonio | - |
dc.contributor.author | Sebastián Suarez, Juan | - |
dc.contributor.author | Soto-Sánchez, Cristina | - |
dc.contributor.author | Garrigós, Javier | - |
dc.contributor.author | Martínez-Alvárez, J.Javier | - |
dc.contributor.author | Ferrández, J. Manuel | - |
dc.contributor.author | Fernández, Eduardo | - |
dc.contributor.other | Departamentos de la UMH::Histología y Anatomía | es_ES |
dc.date.accessioned | 2025-01-23T20:02:06Z | - |
dc.date.available | 2025-01-23T20:02:06Z | - |
dc.date.created | 2020-09-03 | - |
dc.identifier.citation | Int J Neural Syst . 2020 Sep;30(9):2050045. | es_ES |
dc.identifier.issn | 1793-6462 | - |
dc.identifier.issn | 0129-0657 | - |
dc.identifier.uri | https://hdl.handle.net/11000/35180 | - |
dc.description.abstract | Visual neuroprosthesis, that provide electrical stimulation along several sites of the human visual system, constitute a potential tool for vision restoration for the blind. Scientific and technological progress in the fields of neural engineering and artificial vision comes with new theories and tools that, along with the dawn of modern artificial intelligence, constitute a promising framework for the further development of neurotechnology. In the framework of the development of a Cortical Visual Neuroprosthesis for the blind (CORTIVIS), we are now facing the challenge of developing not only computationally powerful tools and flexible approaches that will allow us to provide some degree of functional vision to individuals who are profoundly blind. In this work, we propose a general neuroprosthesis framework composed of several task-oriented and visual encoding modules. We address the development and implementation of computational models of the firing rates of retinal ganglion cells and design a tool - Neurolight - that allows these models to be interfaced with intracortical microelectrodes in order to create electrical stimulation patterns that can evoke useful perceptions. In addition, the developed framework allows the deployment of a diverse array of state-of-the-art deep-learning techniques for task-oriented and general image pre-processing, such as semantic segmentation and object detection in our system's pipeline. To the best of our knowledge, this constitutes the first deep-learning-based system designed to directly interface with the visual brain through an intracortical microelectrode array. We implement the complete pipeline, from obtaining a video stream to developing and deploying task-oriented deep-learning models and predictive models of retinal ganglion cells' encoding of visual inputs under the control of a neurostimulation device able to send electrical train pulses to a microelectrode array implanted at the visual cortex. | es_ES |
dc.format | application/pdf | es_ES |
dc.format.extent | 19 | es_ES |
dc.language.iso | eng | es_ES |
dc.publisher | Word Scientific Publising | es_ES |
dc.rights | info:eu-repo/semantics/closedAccess | es_ES |
dc.rights | Attribution-NonCommercial-NoDerivatives 4.0 Internacional | * |
dc.rights.uri | http://creativecommons.org/licenses/by-nc-nd/4.0/ | * |
dc.subject | vsual neuroprosthesis | es_ES |
dc.subject | artificial vision | es_ES |
dc.subject | computational models | es_ES |
dc.subject | deep learning | es_ES |
dc.subject | neural encoding | es_ES |
dc.title | Neurolight: A Deep Learning Neural Interface for Cortical Visual Prostheses | es_ES |
dc.type | info:eu-repo/semantics/article | es_ES |
dc.relation.publisherversion | 10.1142/S0129065720500458 | es_ES |
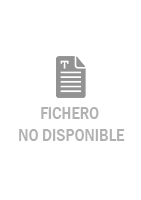
Neurolight-A-Deep-Learning-Neural-Interface-for-Cortical-Visual-Prostheses.pdf
4,91 MB
Adobe PDF
Compartir:
La licencia se describe como: Atribución-NonComercial-NoDerivada 4.0 Internacional.