Por favor, use este identificador para citar o enlazar este ítem:
https://hdl.handle.net/11000/34876
Registro completo de metadatos
Campo DC | Valor | Lengua/Idioma |
---|---|---|
dc.contributor.author | VACA LAMATA, MARTA | - |
dc.contributor.author | Perez Martin, Agustin | - |
dc.contributor.author | Pérez-Torregrosa, Agustín | - |
dc.contributor.other | Departamentos de la UMH::Estudios Económicos y Financieros | es_ES |
dc.date.accessioned | 2025-01-18T08:26:09Z | - |
dc.date.available | 2025-01-18T08:26:09Z | - |
dc.date.created | 2018 | - |
dc.identifier.citation | Journal of Business Research | es_ES |
dc.identifier.issn | 1873-7978 | - |
dc.identifier.uri | https://hdl.handle.net/11000/34876 | - |
dc.description.abstract | Abstract Nowadays, the volume of databases that financial companies manage is so great that it has become necessary to address this problem, and the solution to this can be found in Big Data techniques applied to massive financial datasets for segmenting risk groups. In this paper, the presence of large datasets is approached through the development of some Monte Carlo experiments using known techniques and algorithms. In addition, a linear mixed model (LMM) has been implemented as a new incremental contribution to calculate the credit risk of financial companies. These computational experiments are developed with several combinations of dataset sizes and forms to cover a wide variety of cases. Results reveal that large datasets need Big Data techniques and algorithms that yield faster and unbiased estimators. Big Data can help to extract the value of data and thus better decisions can be made without the runtime component. Through these techniques, there would be less risk for financial companies when predicting which clients will be successful in their payments. Consequently, more people could have access to credit loans. | es_ES |
dc.format | application/pdf | es_ES |
dc.format.extent | 7 | es_ES |
dc.language.iso | eng | es_ES |
dc.publisher | Elsevier | es_ES |
dc.relation.ispartofseries | 89 | es_ES |
dc.rights | info:eu-repo/semantics/openAccess | es_ES |
dc.rights | Attribution-NonCommercial-NoDerivatives 4.0 Internacional | * |
dc.rights.uri | http://creativecommons.org/licenses/by-nc-nd/4.0/ | * |
dc.subject | Credit scoring | es_ES |
dc.subject | Big Data | es_ES |
dc.subject | Monte Carlo | es_ES |
dc.subject | Data mining | es_ES |
dc.subject.other | CDU::3 - Ciencias sociales::33 - Economía | es_ES |
dc.title | Big Data techniques to measure credit banking risk in home equity loans | es_ES |
dc.type | info:eu-repo/semantics/article | es_ES |
dc.relation.publisherversion | https://doi.org/10.1016/j.jbusres.2018.02.008 Get rights and content | es_ES |
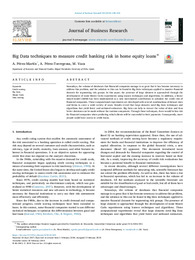
Ver/Abrir:
articulo_publicadogika.pdf
613,88 kB
Adobe PDF
Compartir:
La licencia se describe como: Atribución-NonComercial-NoDerivada 4.0 Internacional.