Please use this identifier to cite or link to this item:
https://hdl.handle.net/11000/34524
Full metadata record
DC Field | Value | Language |
---|---|---|
dc.contributor.author | López García, Miguel | - |
dc.contributor.author | VALERO, SERGIO | - |
dc.contributor.author | Sans, Carlos | - |
dc.contributor.other | Departamentos de la UMH::Ingeniería Mecánica y Energía | es_ES |
dc.date.accessioned | 2025-01-15T19:41:25Z | - |
dc.date.available | 2025-01-15T19:41:25Z | - |
dc.date.created | 2022 | - |
dc.identifier.citation | Electric Power Systems Research | es_ES |
dc.identifier.issn | 1873-2046 | - |
dc.identifier.issn | 0378-7796 | - |
dc.identifier.uri | https://hdl.handle.net/11000/34524 | - |
dc.description.abstract | Electricity demand presents a repetitive pattern following daily, weekly and seasonal patterns. However, factors like temperature or social events tend to disrupt these patterns introducing outlying data that is difficult to forecast. This paper introduces a new methodology to classify special days without any prior knowledge of the database. Simple classification of special days into two or three categories is insufficient as the consumers’ behavior has many shades on these days. However, classifying special days in a wide range of categories required a deep understanding of the consumers’ behavior on different days and periods of the year. The methodology proposed describes an algorithm to automate this classification starting from a simple day-of-the-week classification and branching into as many categories as needed to fit a real database. Categories with similar profiles are merged to avoid overfitting and actual outliers are detected to ensure that no false categories are created. The methodology is developed using data from 2010 to 2017 and tested in three different systems. The benchmark used is the classification used by the Transmission System Operator in Spain and the test show that the proposed methodology provides more accurate results without the need of an expert to develop the classification. | es_ES |
dc.format | application/pdf | es_ES |
dc.format.extent | 9 | es_ES |
dc.language.iso | eng | es_ES |
dc.publisher | Elsevier | es_ES |
dc.relation.ispartofseries | 202 | es_ES |
dc.rights | info:eu-repo/semantics/openAccess | es_ES |
dc.rights | Attribution-NonCommercial-NoDerivatives 4.0 Internacional | * |
dc.rights.uri | http://creativecommons.org/licenses/by-nc-nd/4.0/ | * |
dc.subject | Load forecasting | es_ES |
dc.subject | Power demand | es_ES |
dc.subject | Holidays | es_ES |
dc.subject.other | CDU::6 - Ciencias aplicadas::62 - Ingeniería. Tecnología::621 - Ingeniería mecánica en general. Tecnología nuclear. Electrotecnia. Maquinaria | es_ES |
dc.title | Automatic classification of special days for short-term load forecasting | es_ES |
dc.type | info:eu-repo/semantics/article | es_ES |
dc.relation.publisherversion | https://doi.org/10.1016/j.epsr.2021.107533 | es_ES |
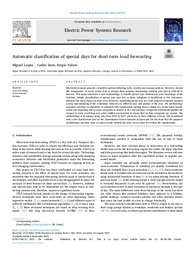
View/Open:
1-s2.0-S0378779621005149-main.pdf
1,31 MB
Adobe PDF
Share: