Por favor, use este identificador para citar o enlazar este ítem:
https://hdl.handle.net/11000/8526
Registro completo de metadatos
Campo DC | Valor | Lengua/Idioma |
---|---|---|
dc.contributor.advisor | Migallón Gomis, Héctor Francisco | - |
dc.contributor.advisor | Martínez Rach, Miguel Onofre | - |
dc.contributor.author | Del Campo Calvo, Francisco Javier | - |
dc.contributor.other | Departamentos de la UMH::Ingeniería de Computadores | es |
dc.date.accessioned | 2021-09-27T15:59:35Z | - |
dc.date.available | 2021-09-27T15:59:35Z | - |
dc.date.created | 2021-07-02 | - |
dc.date.issued | 2021-07-02 | - |
dc.identifier.uri | http://hdl.handle.net/11000/8526 | - |
dc.description.abstract | Este Trabajo de Fin de Grado está estructurado en dos partes, siendo la primera un análisis del rendimiento de tres algoritmos metaheurísticos de optimización discreta: DTSA (Discrete Tree-Seed Algorithm), DJAYA (Discrete Jaya) y DTLBO (Discrete Teaching-Learning-Based Optimization). Para realizar dicho análisis se han implementado los tres algoritmos en lenguaje C y se han probado con 6 problemas del tipo TSP. El otro objetivo del trabajo es el desarrollo y posterior análisis de eficiencia y escalabilidad de diseños paralelos que permitan acelerar la ejecución de los algoritmos metaheurísticos estudiados en equipos de computación de altas prestaciones con arquitectura de memoria compartida. Han sido implementados tres algoritmos paralelos: un enfoque basado en automatizadores, un enfoque basado en subpoblaciones y finalmente un enfoque híbrido, siendo aplicables todos ellos a cada uno de los tres algoritmos metaheurísticos. Estos algoritmos han sido implementados usando OpenMP. El análisis de rendimiento de los algoritmos metaheurísticos ha mostrado que los algoritmos DTSA y DJAYA obtienen resultados que DTLBO para los problemas estudiados, teniendo además DJAYA una velocidad de convergencia ligeramente mejor. Por otra parte, del análisis de eficiencia paralela se ha deducido que el enfoque basado en automatizadores obtiene resultados de eficiencia y escalabilidad mucho más positivos para el algoritmo DTSA que para DJAYA y DTLBO, mientras que el enfoque basado en subpoblaciones ha obtenido unos resultados muy buenos en todos los casos. Finalmente, los algoritmos híbridos no han mostrado una disminución apreciable en la eficiencia y en la escalabilidad respecto del enfoque con subpoblaciones, lo que sumado a su gran versatilidad supone constatar que son un muy buen diseño para aplicar a este tipo de algoritmos. | es |
dc.description.abstract | This Final Degree Project is structured in two parts, the first being a performance analysis of three discrete optimization metaheuristic algorithms: DTSA (Discrete Tree-Seed Algorithm), DJAYA (Discrete Jaya) y DTLBO (Discrete Teaching-Learning-Based Optimization). To perform this analysis, the three algorithms have been implemented in C language and 6 TSP problems have been used to try them. The other aim of this project is to develop parallel designs to speed up the runtime of the studied metaheuristic algorithms in high-performance computing systems with shared memory architecture. Three parallel algorithms have been implemented: an automatic-parallelization-based approach, a subpopulation-based approach, and a hybrid approach, all of them being applicable to each of the three metaheuristic algorithms. These algorithms have been implemented using OpenMP. An efficiency and scalability analysis of the three parallel algorithms has been performed. The performance analysis carried out on the metaheuristic algorithms has proved that DTSA and DJAYA algorithms obtain better results than DTLBO for the studied problems, having DJAYA a slightly better convergence speed than DTSA. On the other hand, the parallel efficiency analysis has shown up that automatic-parallelization-based approach gets much better efficiency and scalability results when applied to DTSA algorithm than when applied to DJAYA and DTLBO, while subpopulation-based approach has obtained very good results in all cases. Finally, the hybrid algorithms have not shown an appreciable decrease in efficiency and scalability in comparison with subpopulation-based algorithms, which added to their great versatility, makes them a very good design for applying to metaheuristic algorithms similar to those studied. | es |
dc.format | application/pdf | es |
dc.format.extent | 116 | es |
dc.language.iso | spa | es |
dc.publisher | Universidad Miguel Hernández de Elche | es |
dc.rights | info:eu-repo/semantics/openAccess | es |
dc.subject | optimización | es |
dc.subject | optimización discreta | es |
dc.subject | algoritmos de optimización | es |
dc.subject | algoritmos heurísticos | es |
dc.subject | algoritmos paralelos | es |
dc.subject.other | CDU::6 - Ciencias aplicadas::62 - Ingeniería. Tecnología | es |
dc.title | Análisis y aceleración de algoritmos metaheurísticos de optimización discreta | es |
dc.type | info:eu-repo/semantics/bachelorThesis | es |
dc.type | info:eu-repo/semantics/bachelorThesis | es |
dc.type | info:eu-repo/semantics/bachelorThesis | es |
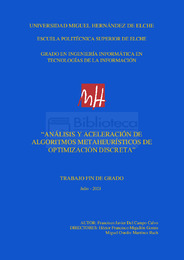
Ver/Abrir:
TFG-Del Campo Calvo, Francisco Javier.pdf
3,7 MB
Adobe PDF
Compartir:
La licencia se describe como: Atribución-NonComercial-NoDerivada 4.0 Internacional.