Por favor, use este identificador para citar o enlazar este ítem:
https://hdl.handle.net/11000/35400
Registro completo de metadatos
Campo DC | Valor | Lengua/Idioma |
---|---|---|
dc.contributor.author | Serrano Rodríguez, Juan Miguel | - |
dc.contributor.author | Navarro Cobacho, Pedro | - |
dc.contributor.author | Ruiz Ramírez, Javier | - |
dc.contributor.author | Palenzuela, Patricia | - |
dc.contributor.author | Lucas Miralles, Manuel | - |
dc.contributor.author | Roca, Lidia | - |
dc.contributor.other | Departamentos de la UMH::Ingeniería Mecánica y Energía | es_ES |
dc.date.accessioned | 2025-01-28T13:46:34Z | - |
dc.date.available | 2025-01-28T13:46:34Z | - |
dc.date.created | 2024 | - |
dc.identifier.citation | Energy | es_ES |
dc.identifier.issn | 1873-6785 | - |
dc.identifier.issn | 0360-5442 | - |
dc.identifier.uri | https://hdl.handle.net/11000/35400 | - |
dc.description.abstract | The efficiency of Concentrated Solar Power (CSP) plants strongly depends on steam condensation temperatures. Current cooling systems, either wet (water-cooled) or dry (air-cooled), present trade-offs. Wet cooling towers (WCT) optimize performance but raise concerns due to substantial water usage, especially in water-scarce prone locations of CSP plants. Dry cooling conserves water but sacrifices efficiency, specially during high ambient temperatures, coinciding with peak electricity demand. A potential compromise is a combined cooling system, integrating wet and dry methods, offering lower water consumption, improved efficiency and flexibility. Incorporating such systems into CSP plants is of considerable interest, aiming to optimize operations under diverse conditions. This research focuses on the first step towards this goal; developing static models for WCTs. Two approaches, Poppe and Artificial Neural Networks (ANN), are developed and thoroughly compared in terms of prediction capabilities, experimental and instrumentation requirements, sensitivity analysis, execution time, implementation and scalability. Both approaches have proven to be reliable, with Poppe providing better results, based on MAPE, for the outlet temperature and water consumption (0.87 % and 3.74 %, respectively) compared to a cascade-forward ANN model (1.82 % and 5.21 %, respectively). However, for the target application, the better execution time favours the use of ANNs. | es_ES |
dc.format | application/pdf | es_ES |
dc.format.extent | 15 | es_ES |
dc.language.iso | eng | es_ES |
dc.publisher | Elsevier | es_ES |
dc.relation.ispartofseries | 305 | es_ES |
dc.rights | info:eu-repo/semantics/openAccess | es_ES |
dc.rights | Attribution-NonCommercial-NoDerivatives 4.0 Internacional | * |
dc.rights.uri | http://creativecommons.org/licenses/by-nc-nd/4.0/ | * |
dc.subject | Concentrated solar power | es_ES |
dc.subject | Cooling system | es_ES |
dc.subject | Modelling | es_ES |
dc.subject | Neural networks | es_ES |
dc.subject | Sensitivity analysis | es_ES |
dc.subject.other | CDU::6 - Ciencias aplicadas::62 - Ingeniería. Tecnología::621 - Ingeniería mecánica en general. Tecnología nuclear. Electrotecnia. Maquinaria | es_ES |
dc.title | Wet cooling tower performance prediction in CSP plants: A comparison between artificial neural networks and Poppe’s model | es_ES |
dc.type | info:eu-repo/semantics/article | es_ES |
dc.relation.publisherversion | https://doi.org/10.1016/j.energy.2024.131844 | es_ES |
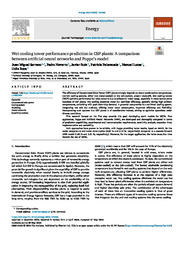
Ver/Abrir:
2024 Energy 303.pdf
2,98 MB
Adobe PDF
Compartir:
La licencia se describe como: Atribución-NonComercial-NoDerivada 4.0 Internacional.