Please use this identifier to cite or link to this item:
https://hdl.handle.net/11000/32252
Full metadata record
DC Field | Value | Language |
---|---|---|
dc.contributor.author | Cezar, Everson | - |
dc.contributor.author | NANNI, MARCOS | - |
dc.contributor.author | Guerrero, César | - |
dc.contributor.author | Silva Junior, Carlos Antonio da | - |
dc.contributor.author | Teixeira Cruciol, Luiz Guilherme | - |
dc.contributor.author | Luiz Chicati, Marcelo | - |
dc.contributor.author | Capristo-Silva, Guilherme Fernando | - |
dc.contributor.other | Departamentos de la UMH::Agroquímica y Medio Ambiente | es_ES |
dc.date.accessioned | 2024-06-03T07:37:29Z | - |
dc.date.available | 2024-06-03T07:37:29Z | - |
dc.date.created | 2019-04-15 | - |
dc.identifier.citation | Geoderma, Volume 340, 15 April 2019, Pages 224-23 | es_ES |
dc.identifier.issn | 0016-7061 | - |
dc.identifier.uri | https://hdl.handle.net/11000/32252 | - |
dc.description.abstract | The development of spectral prediction models for soil attributes has been extensively studied in the last 10 years. However, one of the problems encountered during this period concerns the representativeness of the samples selected for model generation, which are often unable to capture the existing variability in agricultural areas, generating imprecise models. Thus, it is necessary to establish strategies for selecting soil samples, as well as for making them more representative within the model. Considering this, the aim of the present study was to evaluate strategies for soil sample selection and the recalibration of large models using samples from a smaller area, in a process called spiking, and its effect on soil attribute estimations. A total of 425 soil samples were used for the generation of the state models, as well as 200 soil samples from a target site for attribute recalibration and prediction. From these 200 samples, 10 (subset) were selected by different methods for state model recalibration (spiking), and 190 were used in the prediction. Another 5 and 10 copies of the subsets were also used as extra-weight to recalibrate the models. Models spiked with samples located in the center of the spectral space associated with extra-weight (10 copies) showed better accuracy in sand prediction (RPD = 2.20; r2 = 0.80; RMSEP = 71.6 g kg−1). For organic matter, the use of selected samples based on 5 clusters associated with extra-weight (10 copies) slightly improved the RMSEP and RPD in most cases, reaching a maximum value of 6.1 g dm−3 and 1.20, respectively. However, the subsets selected at the target site were not able to indicate the entire variability of the local samples concerning organic matter, damaging the expansion of the recalibrated state models. | es_ES |
dc.format | application/pdf | es_ES |
dc.format.extent | 63 | es_ES |
dc.language.iso | eng | es_ES |
dc.publisher | Elsevier | es_ES |
dc.rights | info:eu-repo/semantics/openAccess | es_ES |
dc.rights | Attribution-NonCommercial-NoDerivatives 4.0 Internacional | * |
dc.rights.uri | http://creativecommons.org/licenses/by-nc-nd/4.0/ | * |
dc.subject | Spiking | es_ES |
dc.subject | Sand | es_ES |
dc.subject | Organic matter | es_ES |
dc.subject | Spectroradiometry | es_ES |
dc.subject.other | CDU::5 - Ciencias puras y naturales | es_ES |
dc.title | Organic matter and sand estimates by spectroradiometry: Strategies for the development of models with applicability at a local scale | es_ES |
dc.type | info:eu-repo/semantics/article | es_ES |
dc.relation.publisherversion | https://doi.org/10.1016/j.geoderma.2019.01.021 | es_ES |
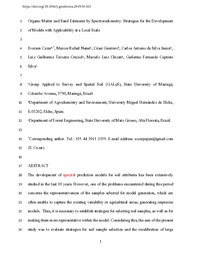
View/Open:
Organic Matter and Sand Estimates by Spectroradiometry.pdf
1,39 MB
Adobe PDF
Share: