Please use this identifier to cite or link to this item:
https://hdl.handle.net/11000/31253
Full metadata record
DC Field | Value | Language |
---|---|---|
dc.contributor.author | Badesa, Francisco Javier | - |
dc.contributor.author | Garcia-Aracil, Nicolas | - |
dc.contributor.author | Catalán, José María | - |
dc.contributor.author | Zollo, Loredana | - |
dc.contributor.author | Pagliara, Silvio Marcello | - |
dc.contributor.author | Sterzi, Silvia | - |
dc.contributor.author | Lauretti, Clemente | - |
dc.contributor.author | Cordella, Francesca | - |
dc.contributor.author | Crea, Simona | - |
dc.contributor.author | Ciancio, Anna Lisa | - |
dc.contributor.author | Trigili, Emilio | - |
dc.contributor.author | Vitiello, Nicola | - |
dc.contributor.other | Departamentos de la UMH::Ingeniería de Sistemas y Automática | es_ES |
dc.date.accessioned | 2024-02-07T17:53:32Z | - |
dc.date.available | 2024-02-07T17:53:32Z | - |
dc.date.created | 2018 | - |
dc.identifier.citation | Frontiers in Neurorobotics Volume 12 - 2018 | es_ES |
dc.identifier.issn | 1662-5218 | - |
dc.identifier.uri | https://hdl.handle.net/11000/31253 | - |
dc.description.abstract | The reference joint position of upper-limb exoskeletons is typically obtained by means of Cartesian motion planners and inverse kinematics algorithms with the inverse Jacobian; this approach allows exploiting the available Degrees of Freedom (i.e. DoFs) of the robot kinematic chain to achieve the desired end-effector pose; however, if used to operate non-redundant exoskeletons, it does not ensure that anthropomorphic criteria are satisfied in the whole human-robot workspace. This paper proposes a motion planning system, based on Learning by Demonstration, for upper-limb exoskeletons that allow successfully assisting patients during Activities of Daily Living (ADLs) in unstructured environment, while ensuring that anthropomorphic criteria are satisfied in the whole human-robot workspace. The motion planning system combines Learning by Demonstration with the computation of Dynamic Motion Primitives and machine learning techniques to construct task- and patient-specific joint trajectories based on the learnt trajectories. System validation was carried out in simulation and in a real setting with a 4- DoF upper-limb exoskeleton, a 5-DoF wrist-hand exoskeleton and four patients with Limb Girdle Muscular Dystrophy. Validation was addressed to (i) compare the performance of the proposed motion planning with traditional methods; (ii) assess the generalization capabilities of the proposed method with respect to the environment variability. Three ADLs were chosen to validate the system: drinking, pouring and lifting a light sphere. The achieved results showed a 100% success rate in the task fulfillment, with a high level of generalization with respect to the environment variability. Moreover, an anthropomorphic configuration of the exoskeleton is always ensured. | es_ES |
dc.format | application/pdf | es_ES |
dc.format.extent | 14 | es_ES |
dc.language.iso | eng | es_ES |
dc.publisher | Frontiers Media | es_ES |
dc.rights | info:eu-repo/semantics/openAccess | es_ES |
dc.rights | Attribution-NonCommercial-NoDerivatives 4.0 Internacional | * |
dc.rights.uri | http://creativecommons.org/licenses/by-nc-nd/4.0/ | * |
dc.subject | motion planning | es_ES |
dc.subject | machine learning | es_ES |
dc.subject | learning by demonstration | es_ES |
dc.subject | dynamics movement primitives | es_ES |
dc.subject | assistive robotics | es_ES |
dc.title | Learning by Demonstration for Motion Planning of Upper-Limb Exoskeletons | es_ES |
dc.type | info:eu-repo/semantics/article | es_ES |
dc.relation.publisherversion | https://doi.org/10.3389/fnbot.2018.00005 | es_ES |
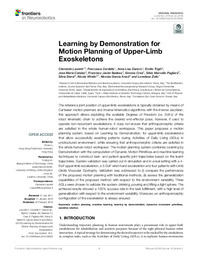
View/Open:
2018_frontiers.pdf
5,06 MB
Adobe PDF
Share: