Please use this identifier to cite or link to this item:
https://hdl.handle.net/11000/30612
Full metadata record
DC Field | Value | Language |
---|---|---|
dc.contributor.author | Chaquet Ulldemolins, Jacobo | - |
dc.contributor.author | Gimeno Blanes, Francisco Javier | - |
dc.contributor.author | Moral-Rubio, Santiago | - |
dc.contributor.author | Muñoz-Romero, Sergio | - |
dc.contributor.author | Rojo-Álvarez, José Luis | - |
dc.contributor.other | Departamentos de la UMH::Ingeniería de Comunicaciones | es_ES |
dc.date.accessioned | 2024-01-24T11:30:58Z | - |
dc.date.available | 2024-01-24T11:30:58Z | - |
dc.date.created | 2022-04 | - |
dc.identifier.citation | Applied Sciences Volume 12 Issue 7 (2022) | es_ES |
dc.identifier.issn | 2076-3417 | - |
dc.identifier.uri | https://hdl.handle.net/11000/30612 | - |
dc.description.abstract | Artificial intelligence (AI) has recently intensified in the global economy due to the great competence that it has demonstrated for analysis and modeling in many disciplines. This situation is accelerating the shift towards a more automated society, where these new techniques can be consolidated as a valid tool to face the difficult challenge of credit fraud detection (CFD). However, tight regulations do not make it easy for financial entities to comply with them while using modern techniques. From a methodological perspective, autoencoders have demonstrated their effectiveness in discovering nonlinear features across several problem domains. However, autoencoders are opaque and often seen as black boxes. In this work, we propose an interpretable and agnostic methodology for CFD. This type of approach allows a double advantage: on the one hand, it can be applied together with any machine learning (ML) technique, and on the other hand, it offers the necessary traceability between inputs and outputs, hence escaping from the black-box model. We first applied the state-of-the-art feature selection technique defined in the companion paper. Second, we proposed a novel technique, based on autoencoders, capable of evaluating the relationship among input and output of a sophisticated ML model for each and every one of the samples that are submitted to the analysis, through a single transaction-level explanation (STE) approach. This technique allows each instance to be analyzed individually by applying small fluctuations of the input space and evaluating how it is triggered in the output, thereby shedding light on the underlying dynamics of the model. Based on this, an individualized transaction ranking (ITR) can be formulated, leveraging on the contributions of each feature through STE. These rankings represent a close estimate of the most important features playing a role in the decision process. The results obtained in this work were consistent with previous published papers, and showed that certain features, such as living beyond means, lack or absence of transaction trail, and car loans, have strong influence on the model outcome. Additionally, this proposal using the latent space outperformed, in terms of accuracy, our previous results, which already improved prior published papers, by 5.5% and 1.5% for the datasets under study, from a baseline of 76% and 93%. The contribution of this paper is twofold, as far as a new outperforming CFD classification model is presented, and at the same time, we developed a novel methodology, applicable across classification techniques, that allows to breach black-box models, erasingthe dependencies and, eventually, undesirable biases. We conclude that it is possible to develop an effective, individualized, unbiased, and traceable ML technique, not only to comply with regulations, but also to be able to cope with transaction-level inquiries from clients and authorities | es_ES |
dc.format | application/pdf | es_ES |
dc.format.extent | 28 | es_ES |
dc.language.iso | eng | es_ES |
dc.publisher | MDPI | es_ES |
dc.rights | info:eu-repo/semantics/openAccess | es_ES |
dc.rights | Attribution-NonCommercial-NoDerivatives 4.0 Internacional | * |
dc.rights.uri | http://creativecommons.org/licenses/by-nc-nd/4.0/ | * |
dc.subject | credit fraud detection | es_ES |
dc.subject | explainable machine learning | es_ES |
dc.subject | interpretability | es_ES |
dc.subject | autoencoders | es_ES |
dc.subject.other | CDU::6 - Ciencias aplicadas::62 - Ingeniería. Tecnología | es_ES |
dc.title | On the Black-Box Challenge for Fraud Detection Using Machine Learning (II): Nonlinear Analysis through Interpretable Autoencoders | es_ES |
dc.type | info:eu-repo/semantics/article | es_ES |
dc.relation.publisherversion | https://doi.org/10.3390/app12073328 | es_ES |
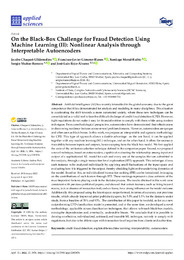
View/Open:
220411 On the Black-Box Challenge for Fraud Detection (II) - applsci-12-03856.pdf
1,47 MB
Adobe PDF
Share: