Por favor, use este identificador para citar o enlazar este ítem:
https://hdl.handle.net/11000/30607
Registro completo de metadatos
Campo DC | Valor | Lengua/Idioma |
---|---|---|
dc.contributor.author | Vayas-Ortega, Germania | - |
dc.contributor.author | Soguero-Ruiz, Cristina | - |
dc.contributor.author | Rodríguez Ibáñez, Margarita | - |
dc.contributor.author | Rojo-Álvarez, José Luis | - |
dc.contributor.author | Gimeno Blanes, Francisco Javier | - |
dc.contributor.other | Departamentos de la UMH::Ingeniería de Comunicaciones | es_ES |
dc.date.accessioned | 2024-01-24T11:25:54Z | - |
dc.date.available | 2024-01-24T11:25:54Z | - |
dc.date.created | 2020-07 | - |
dc.identifier.citation | Applied Sciences Volume 10 Issue 15 (2020) | es_ES |
dc.identifier.issn | 2076-3417 | - |
dc.identifier.uri | https://hdl.handle.net/11000/30607 | - |
dc.description.abstract | The search for an unbiased company valuation method to reduce uncertainty, whether or not it is automatic, has been a relevant topic in social sciences and business development for decades. Many methods have been described in the literature, but consensus has not been reached. In the companion paper we aimed to review the assessment capabilities of traditional company valuation model, based on company’s intrinsic value using the Discounted Cash Flow (DCF). In this paper, we capitalized on the potential of exogenous information combined with Machine Learning (ML) techniques. To do so, we performed an extensive analysis to evaluate the predictive capabilities with up to 18 different ML techniques. Endogenous variables (features) related to value creation (DCF) were proved to be crucial elements for the models, while the incorporation of exogenous, industry/country specific ones, incrementally improves the ML performance. Bagging Trees, Supported Vector Machine Regression, Gaussian Process Regression methods consistently provided the best results. We concluded that an unbiased model can be created based on endogenous and exogenous information to build a reference framework, to price and benchmark Enterprise Value for valuation and credit risk assessment. | es_ES |
dc.format | application/pdf | es_ES |
dc.format.extent | 17 | es_ES |
dc.language.iso | eng | es_ES |
dc.publisher | MDPI | es_ES |
dc.rights | info:eu-repo/semantics/openAccess | es_ES |
dc.rights | Attribution-NonCommercial-NoDerivatives 4.0 Internacional | * |
dc.rights.uri | http://creativecommons.org/licenses/by-nc-nd/4.0/ | * |
dc.subject | company valuation | es_ES |
dc.subject | enterprise value | es_ES |
dc.subject | machine learning | es_ES |
dc.subject | feature selection | es_ES |
dc.subject | supervised techniques | es_ES |
dc.subject | supported vector machine | es_ES |
dc.subject | decision trees | es_ES |
dc.subject | boosting trees | es_ES |
dc.title | On the Differential Analysis of Enterprise Valuation Methods as a Guideline for Unlisted Companies Assessment (II): Applying Machine-Learning Techniques for Unbiased Enterprise Value Assessment | es_ES |
dc.type | info:eu-repo/semantics/article | es_ES |
dc.relation.publisherversion | https://doi.org/ 10.3390/app10155334 | es_ES |
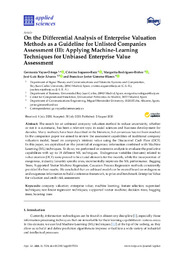
Ver/Abrir:
200802 On the Differential Analysis of Enterprise Valuation 2 - applsci-10-05334.pdf
1,8 MB
Adobe PDF
Compartir:
La licencia se describe como: Atribución-NonComercial-NoDerivada 4.0 Internacional.