Por favor, use este identificador para citar o enlazar este ítem:
https://hdl.handle.net/11000/30592
Registro completo de metadatos
Campo DC | Valor | Lengua/Idioma |
---|---|---|
dc.contributor.author | Bleijendaal, Hidde | - |
dc.contributor.author | Melgarejo Meseguer, Francisco Manuel | - |
dc.contributor.author | Gimeno Blanes, Francisco Javier | - |
dc.contributor.author | Gimeno Blanes, Juan | - |
dc.contributor.author | Pinto, Yigal M. | - |
dc.contributor.author | Marquering, Henk | - |
dc.contributor.author | Zwinderman, Aeilko H | - |
dc.contributor.other | Departamentos de la UMH::Ingeniería de Comunicaciones | es_ES |
dc.date.accessioned | 2024-01-24T08:13:30Z | - |
dc.date.available | 2024-01-24T08:13:30Z | - |
dc.date.created | 2020-09 | - |
dc.identifier.citation | Heart Rhythm Volume 18, Issue 1, January (2021) | es_ES |
dc.identifier.issn | 1547-5271 | - |
dc.identifier.uri | https://hdl.handle.net/11000/30592 | - |
dc.description.abstract | Background Phospholamban (PLN) p.Arg14del mutation carriers are known to develop dilated and/or arrhythmogenic cardiomyopathy, and typical electrocardiographic (ECG) features have been identified for diagnosis. Machine learning is a powerful tool used in ECG analysis and has shown to outperform cardiologists. Objectives We aimed to develop machine learning and deep learning models to diagnose PLN p.Arg14del cardiomyopathy using ECGs and evaluate their accuracy compared to an expert cardiologist. Methods We included 155 adult PLN mutation carriers and 155 age- and sex-matched control subjects. Twenty-one PLN mutation carriers (13.4%) were classified as symptomatic (symptoms of heart failure or malignant ventricular arrhythmias). The data set was split into training and testing sets using 4-fold cross-validation. Multiple models were developed to discriminate between PLN mutation carriers and control subjects. For comparison, expert cardiologists classified the same data set. The best performing models were validated using an external PLN p.Arg14del mutation carrier data set from Murcia, Spain (n = 50). We applied occlusion maps to visualize the most contributing ECG regions. Results In terms of specificity, expert cardiologists (0.99) outperformed all models (range 0.53–0.81). In terms of accuracy and sensitivity, experts (0.28 and 0.64) were outperformed by all models (sensitivity range 0.65–0.81). T-wave morphology was most important for classification of PLN p.Arg14del carriers. External validation showed comparable results, with the best model outperforming experts. Conclusion This study shows that machine learning can outperform experienced cardiologists in the diagnosis of PLN p.Arg14del cardiomyopathy and suggests that the shape of the T wave is of added importance to this diagnosis. | es_ES |
dc.format | application/pdf | es_ES |
dc.format.extent | 9 | es_ES |
dc.language.iso | eng | es_ES |
dc.publisher | Elsevier | es_ES |
dc.rights | info:eu-repo/semantics/openAccess | es_ES |
dc.rights.uri | http://creativecommons.org/licenses/by-nc-nd/4.0/ | * |
dc.subject | Cardiomyopathy | es_ES |
dc.subject | Deep learning | es_ES |
dc.subject | ECG analysis | es_ES |
dc.subject | Genetic heart disease | es_ES |
dc.subject | Machine learning | es_ES |
dc.subject | Phospholamban | es_ES |
dc.subject.other | CDU::6 - Ciencias aplicadas::62 - Ingeniería. Tecnología | es_ES |
dc.title | Computer versus cardiologist: Is a machine learning algorithm able to outperform an expert in diagnosing a phospholamban p.Arg14del mutation on the electrocardiogram? | es_ES |
dc.type | info:eu-repo/semantics/article | es_ES |
dc.relation.publisherversion | https://doi.org/10.1016/j.hrthm.2020.08.021 | es_ES |
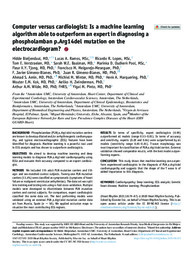
Ver/Abrir:
210101 Computer versus cardiologist_ Is a machine learning.pdf
1,77 MB
Adobe PDF
Compartir:
La licencia se describe como: Atribución-NonComercial-NoDerivada 4.0 Internacional.