Por favor, use este identificador para citar o enlazar este ítem:
https://hdl.handle.net/11000/31573
Registro completo de metadatos
Campo DC | Valor | Lengua/Idioma |
---|---|---|
dc.contributor.author | Soler Gil, Francisco José | - |
dc.contributor.author | Jiménez, Luis M. | - |
dc.contributor.author | Valiente, David | - |
dc.contributor.author | Paya, Luis | - |
dc.contributor.author | Reinoso, Oscar | - |
dc.contributor.other | Departamentos de la UMH::Ingeniería de Sistemas y Automática | es_ES |
dc.date.accessioned | 2024-02-28T11:27:08Z | - |
dc.date.available | 2024-02-28T11:27:08Z | - |
dc.date.created | 2023-11 | - |
dc.identifier.citation | Proceedings of the 20th International Conference on Informatics in Control, Automation and Robotics - (Volume 1) | es_ES |
dc.identifier.isbn | 978-989-758-670-5 | - |
dc.identifier.issn | 2184-2809 | - |
dc.identifier.uri | https://hdl.handle.net/11000/31573 | - |
dc.description.abstract | Nowadays neural networks are widely used for segmentation tasks and there is a belief that these approaches are synonymous of advances and improvements. This article aims to compare the performance of a neural network, trained in our previous work, and an algorithm which is specifically designed for the segmentation of reticular structures. As shown in this paper, in certain cases it is feasible to use conventional techniques outside the paradigm of artificial intelligence achieving the same performance. To prove this, in this article a quantitative and qualitative comparative analysis is carried out between an ad hoc algorithm for segmenting reticular structures and the model of neural network that provided the best results in our previous work in this task. Established techniques such as Random Sample Consensus (RANSAC) and region growing have been used to implement the proposed algorithm. For the quantitative analysis, standard metrics such as precision, recall and f1-score are used. These metrics will be calculated with a self-generated dataset, consisting of a thousand point clouds that were generated automatically in the previous work. The studied algorithm is tailor-made for this database. For reproducibility, code and datasets are provided at https://github.com/Urwik/ rrss grnd filter.git. | es_ES |
dc.format | application/pdf | es_ES |
dc.format.extent | 31 | es_ES |
dc.language.iso | eng | es_ES |
dc.publisher | INSTICC - Institute for Systems and Technologies of Information, Control and Communication | es_ES |
dc.relation.ispartof | Proceedings of the 20th International Conference on Informatics in Control, Automation and Robotics Volume 1 | es_ES |
dc.rights | info:eu-repo/semantics/openAccess | es_ES |
dc.rights.uri | http://creativecommons.org/licenses/by-nc-nd/4.0/ | * |
dc.subject | Plane Segmentation | es_ES |
dc.subject | Point Clouds | es_ES |
dc.subject | Region Growing | es_ES |
dc.subject | RANSAC | es_ES |
dc.subject | Neural Networks | es_ES |
dc.subject | Climbing Robots | es_ES |
dc.subject.other | CDU::6 - Ciencias aplicadas::62 - Ingeniería. Tecnología | es_ES |
dc.title | Comparative Analysis of Segmentation Techniques for Reticular Structures | es_ES |
dc.type | info:eu-repo/semantics/article | es_ES |
dc.relation.publisherversion | https://doi.org/10.5220/0000168300003543 | es_ES |
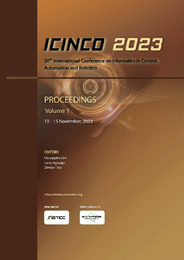
Ver/Abrir:
2023_ICINCO_Estructuras (2) (1)-222.pdf
9,33 MB
Adobe PDF
Compartir:
La licencia se describe como: Atribución-NonComercial-NoDerivada 4.0 Internacional.