Por favor, use este identificador para citar o enlazar este ítem:
https://hdl.handle.net/11000/30602
Registro completo de metadatos
Campo DC | Valor | Lengua/Idioma |
---|---|---|
dc.contributor.author | Rodríguez Ibáñez, Margarita | - |
dc.contributor.author | Muñoz-Romero, Sergio | - |
dc.contributor.author | Soguero-Ruiz, Cristina | - |
dc.contributor.author | Gimeno Blanes, Francisco Javier | - |
dc.contributor.author | Rojo-Álvarez, José Luis | - |
dc.contributor.other | Departamentos de la UMH::Ingeniería de Comunicaciones | es_ES |
dc.date.accessioned | 2024-01-24T11:19:51Z | - |
dc.date.available | 2024-01-24T11:19:51Z | - |
dc.date.created | 2019-06 | - |
dc.identifier.citation | IEEE Access v.7 (2019) | es_ES |
dc.identifier.issn | 2169-3536 | - |
dc.identifier.uri | https://hdl.handle.net/11000/30602 | - |
dc.description.abstract | With the emergence of information and communication technologies, a large amount of data has turned available for the organizations, which creates expectations on their value and content for management purposes. However, the exploratory analysis of available organizational data based on emerging Big Data technologies are still developing in terms of operative tools for solid and interpretable data description. In this work, we addressed the exploratory analysis of organization databases at early stages where little quantitative information is available about their efficiency. Categorical and metric single-variable tests are proposed and formalized in order to provide a mass criterion to identify regions in forms with clusters of significant variables. Bootstrap resampling techniques are used to provide nonparametric criteria in order to establish easy-to-use statistical tests, so that single-variable tests are represented each on a visual and quantitative statistical plot, whereas all the variables in a given form are jointly visualized in the so-called chromosome plots. More detailed profile plots offer deep comparison knowledge for categorical variables across the organization physical and functional structures, while histogram plots for numerical variables incorporate the statistical significance of the variables under study for preselected Pareto groups. Performance grouping is addressed by identifying two or three groups according to some representative empirical distribution of some convenient grouping feature. The method is applied to perform a Big-Data exploratory analysis on the follow-up forms of Spanish Red Cross, based on the number of interventions and on a by-record basis. Results showed that a simple one-variable blind-knowledge exploratory Big-Data analysis, as the one developed in this paper, offers unbiased comparative graphical and numerical information that characterize organizational dynamics in terms of applied resources, available capacities | es_ES |
dc.format | application/pdf | es_ES |
dc.format.extent | 14 | es_ES |
dc.language.iso | eng | es_ES |
dc.publisher | Institute of Electrical and Electronics Engineers | es_ES |
dc.rights | info:eu-repo/semantics/openAccess | es_ES |
dc.rights | Attribution-NonCommercial-NoDerivatives 4.0 Internacional | * |
dc.rights.uri | http://creativecommons.org/licenses/by-nc-nd/4.0/ | * |
dc.subject | Organizations | es_ES |
dc.subject | Databases | es_ES |
dc.subject | Big Data | es_ES |
dc.subject | Data mining | es_ES |
dc.subject | Tools | es_ES |
dc.subject | Measurement | es_ES |
dc.subject | Project management | es_ES |
dc.subject.other | CDU::6 - Ciencias aplicadas::62 - Ingeniería. Tecnología | es_ES |
dc.title | Towards Organization Management Using Exploratory Screening and Big Data Tests: A Case Study of the Spanish Red Cross | es_ES |
dc.type | info:eu-repo/semantics/article | es_ES |
dc.relation.publisherversion | https://doi.org/10.1109/ACCESS.2019.2923533 | es_ES |
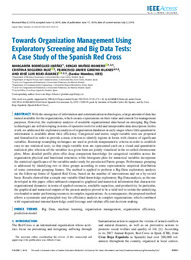
Ver/Abrir:
190701 Towards Organization Management Using Exploratory Screening and Big Data Tests - A Case Study of the Spanish Red Cross.pdf
7,03 MB
Adobe PDF
Compartir:
La licencia se describe como: Atribución-NonComercial-NoDerivada 4.0 Internacional.