Por favor, use este identificador para citar o enlazar este ítem:
https://hdl.handle.net/11000/30550
Registro completo de metadatos
Campo DC | Valor | Lengua/Idioma |
---|---|---|
dc.contributor.author | Bosch-Romeu, Raquel | - |
dc.contributor.author | Librero, Julian | - |
dc.contributor.author | Senent Valero, Marina | - |
dc.contributor.author | Sanfeliu-Alonso, Maria Carmen | - |
dc.contributor.author | Salinas-Serrano, Jose Maria | - |
dc.contributor.author | Fores Martos, Jaume | - |
dc.contributor.author | Suay-Garcia, Beatriz | - |
dc.contributor.author | Climent, Joan | - |
dc.contributor.author | Falco, Antonio | - |
dc.contributor.author | Pastor-Valero, Maria | - |
dc.contributor.other | Departamentos de la UMH::Salud Pública, Historia de la Ciencia y Ginecología | es_ES |
dc.date.accessioned | 2024-01-22T17:40:25Z | - |
dc.date.available | 2024-01-22T17:40:25Z | - |
dc.date.created | 2023-01 | - |
dc.identifier.uri | https://hdl.handle.net/11000/30550 | - |
dc.description.abstract | Background: One of the main drawbacks in constructing a classification model is that some or all of the covariates are categorical variables. Classical methods either assign labels to each output of a categorical variable or are summarised measures (frequencies and percentages), which can be interpreted as probabilities. Methods: We adopted a novel mathematical procedure to construct a classification model from categorical variables based on a non-classical probability approach. More specifically, we codified the variables following the categorical data representation from the Discriminant Correspondence Analysis before constructing a non-classical probability matrix system that represents an entangled system of dependent-independent variables. We then developed a disentangled procedure to obtain an empirical density function for each representative class (minimum of two classes). Finally, we constructed our classification model using the density functions. Results: We applied the proposed procedure to build a classification model of the malignancy of Solitary Pulmonary Nodule (SPN) after five years of follow up using routine clinical data. First, with 2/3 (270) of the sample of 404 patients with SPN, we constructed the classification model, and then validated it with the remaining 1/3(134) we validated it. We tested the procedure’s stability by repeating the analysis randomly 1000 times. We obtained a model accuracy of 0.74, an F1 score of 0.58, a Cohen’s Kappa value of 0.41 and a Matthews Correlation Coefficient of 0.45. Finally, the area under the ROC curve was 0.86. Conclusion: The proposed procedure provides a machine learning classification model with an acceptable performance of a classification model of solitary pulmonary nodule malignancy constructed from routine clinical data and mainly composed of categorical variables. It provides an acceptable performance, which could be used by clinicians as a tool to classify SPN malignancy in routine clinical practice. | es_ES |
dc.format | application/pdf | es_ES |
dc.format.extent | 27 | es_ES |
dc.language.iso | eng | es_ES |
dc.rights | info:eu-repo/semantics/openAccess | es_ES |
dc.rights | Attribution-NonCommercial-NoDerivatives 4.0 Internacional | * |
dc.rights.uri | http://creativecommons.org/licenses/by-nc-nd/4.0/ | * |
dc.subject | Classiffication methods | es_ES |
dc.subject | non classical probabilities | es_ES |
dc.subject | solitary pulmonary nodule | es_ES |
dc.subject.other | CDU::6 - Ciencias aplicadas::61 - Medicina | es_ES |
dc.title | A novel approach to learning through categorical variables applicable to the classification of solitary pulmonary nodule malignancy | es_ES |
dc.type | info:eu-repo/semantics/article | es_ES |
dc.relation.publisherversion | https://doi.org/10.21203/rs.3.rs-2502360/v1 | es_ES |
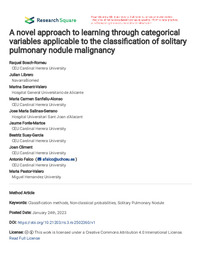
Ver/Abrir:
18-v1_covered_fb50faaf-0e35-4f7c-8796-f5461b050739 (1).pdf
416,63 kB
Adobe PDF
Compartir:
La licencia se describe como: Atribución-NonComercial-NoDerivada 4.0 Internacional.