Por favor, use este identificador para citar o enlazar este ítem:
https://hdl.handle.net/11000/36487
Registro completo de metadatos
Campo DC | Valor | Lengua/Idioma |
---|---|---|
dc.contributor.advisor | Rocamora, Carmen | - |
dc.contributor.advisor | Puerto Molina, Herminia | - |
dc.contributor.author | Codes Alcaraz, Ana María | - |
dc.contributor.other | Departamentos de la UMH::Ingeniería | es_ES |
dc.date.accessioned | 2025-04-14T07:33:33Z | - |
dc.date.available | 2025-04-14T07:33:33Z | - |
dc.date.created | 2025-01 | - |
dc.identifier.uri | https://hdl.handle.net/11000/36487 | - |
dc.description | Programa de Doctorado en Recursos y Tecnologías Agrarias, Agroambientales y Alimentarias | es_ES |
dc.description.abstract | This PhD thesis explores two innovative applications of remote sensing with unmanned aerial vehicles (UAVs) and deep learning techniques. The first study focuses on the traditional irrigation system of the Vega Baja del Segura (Spain), where large amounts of floating waste accumulate affecting irrigation performance and generating serious environmental problems. A dataset was created with 477 images of floating plastic items in different environments and was used for training an algorithm based on YOLOv5s to detect and classify floating debris from UAV images. The trained model achieved a mean average precision (mAP) of 96.9% and the detection speed was 81.7 ms. Overhead photographs were taken with an unmanned aerial vehicle at strategic points of the river and channels, and its automatic count of floating objects was compared with their manual count. The automatic count significantly reduced analysis time and both methods showed good agreement, confirming that water bottles were the most abundant (95%) type of floating waste. This approach provides a reliable tool to monitor debris accumulation, supporting basin authorities in the evaluation of corrective measures to mitigate plastic pollution. The second study focuses on yield estimation in a vineyard located in the Vinalopó bagged table grape production area. A YOLOv7x-based algorithm was trained using UAV imagery to estimate the number of grape clusters. This model accurately predicted the number of grape clusters, with an R2 value of 0.64 and a root mean square error (RMSE) of 0.78 bunches vine−1. Additionally, data from Sentinel-2 and PlanteScope satellites were used to calculate different vegetation indices: Normalized Difference Vegetation Index (NDVI), Soil Adjusted Vegetation Index (SAVI), Enhanced Vegetation Index (EVI), Green Normalized Difference Vegetation Index (GNDVI), Modified Soil Adjusted Vegetation Index (MSAVI), Modified Triangular Vegetation Index (MTVI), Normalized Difference Water Index (NDWI), Optimized Soil Adjusted Vegetation Index (OSAVI), and Simple Ratio Index (SR).. The vegetation indices values showed limited correlation with yield parameters (R2<0.23), as these indices mainly reflect vegetative vigor rather than specific yield attributes. However, the integration of multispectral satellite data, high-resolution UAV imagery, and advanced sensing models offers a robust framework to improve vineyard management and promote more sustainable and efficient agricultural practices. Collectively, these studies highlight the transformative potential of UAVs and AI to to address significant challenges in environmental and agricultural management | es_ES |
dc.format | application/pdf | es_ES |
dc.format.extent | 105 | es_ES |
dc.language.iso | spa | es_ES |
dc.publisher | Universidad Miguel Hernández | es_ES |
dc.rights | info:eu-repo/semantics/openAccess | es_ES |
dc.rights | Attribution-NonCommercial-NoDerivatives 4.0 Internacional | * |
dc.rights.uri | http://creativecommons.org/licenses/by-nc-nd/4.0/ | * |
dc.subject | Residuos plásticos | es_ES |
dc.subject | Teledetección | es_ES |
dc.subject | PlanetScope | es_ES |
dc.subject | Vehículo aéreo no tripulado | es_ES |
dc.subject | YOLO | es_ES |
dc.title | Teledetección con vehículos aéreos no tripulados y técnicas de aprendizaje profundo para la gestión agrícola. Aplicación a dos casos de estudio en la provincia de Alicante | es_ES |
dc.type | info:eu-repo/semantics/doctoralThesis | es_ES |
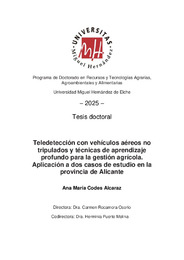
Ver/Abrir:
TD Codes Alcaraz, Ana María.pdf
9,36 MB
Adobe PDF
Compartir:
La licencia se describe como: Atribución-NonComercial-NoDerivada 4.0 Internacional.