Please use this identifier to cite or link to this item:
https://hdl.handle.net/11000/33170
Full metadata record
DC Field | Value | Language |
---|---|---|
dc.contributor.advisor | Rabasa, Alejandro | - |
dc.contributor.advisor | Polotskaya, Kristina | - |
dc.contributor.author | Marco Navarro, Rubén | - |
dc.date.accessioned | 2024-09-17T08:58:52Z | - |
dc.date.available | 2024-09-17T08:58:52Z | - |
dc.date.created | 2024-06 | - |
dc.identifier.uri | https://hdl.handle.net/11000/33170 | - |
dc.description | Especialidad: Informática | es_ES |
dc.description.abstract | El abandono y el fracaso escolar son problemas con un impacto muy negativo sobre la sociedad y sobre el alumno. Tener la capacidad de predecirlos con la suficiente antelación es importante para poder tomar medidas orientadas hacia su prevención, y en ese aspecto los modelos de aprendizaje automático han demostrado ser de gran utilidad. En este trabajo se revisan propuestas recientes encontradas en la literatura científica con el objetivo de arrojar luz sobre qué modelos resultan más apropiados para la predicción del fracaso y el abandono escolar y qué variables poseen un mayor poder predictivo. Los resultados sugieren que los modelos basados en redes neuronales y random forest obtienen la mejor precisión, aunque la variabilidad en el uso de los algoritmos y en las condiciones de entrenamiento impiden afirmar esta tesis con la rotundidad deseada. Se extrae también de la revisión que el balanceo de los datos durante el preprocesamiento incide positiva y significativamente en el rendimiento de los algoritmos y que las variables con mayor poder predictivo son aquellas relacionadas con el ámbito académico | es_ES |
dc.description.abstract | School dropout and failure are problems with a highly negative impact on society and the students. The ability to predict them is important in order to take measures aimed at their prevention, and in this regard, machine learning models have proven to be very useful. This paper reviews recent proposals found in the scientific literature with the aim of shedding light on which models are most appropriate for predicting school failure and dropout and which variables have the greatest predictive power. The results suggest that models based on neural networks and random forests achieve the best accuracy, although the variability in the use of algorithms and training conditions prevents this thesis from being stated with the desired firmness. The review also reveals that data balancing during preprocessing positively and significantly affects the performance of the algorithms and that the variables with the greatest predictive power are those related to the academic domain | es_ES |
dc.format | application/pdf | es_ES |
dc.format.extent | 33 | es_ES |
dc.language.iso | spa | es_ES |
dc.publisher | Universidad Miguel Hernández | es_ES |
dc.rights | info:eu-repo/semantics/openAccess | es_ES |
dc.rights.uri | http://creativecommons.org/licenses/by-nc-nd/4.0/ | * |
dc.subject | Abandono escolar | es_ES |
dc.subject | Abandono escolar | es_ES |
dc.subject | Fracaso académico | es_ES |
dc.subject | Predicción | es_ES |
dc.subject | Aprendizaje automático | es_ES |
dc.subject | Revisión | es_ES |
dc.subject.other | CDU::3 - Ciencias sociales::37 - Educación. Enseñanza. Formación. Tiempo libre | es_ES |
dc.title | Modelos y técnicas para predecir el abandono y el fracaso escolar | es_ES |
dc.type | info:eu-repo/semantics/masterThesis | es_ES |
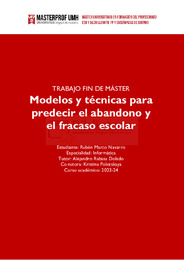
View/Open:
TFM Marco Navarro, Rubén.pdf
430,43 kB
Adobe PDF
Share: