Por favor, use este identificador para citar o enlazar este ítem:
https://hdl.handle.net/11000/35050
Registro completo de metadatos
Campo DC | Valor | Lengua/Idioma |
---|---|---|
dc.contributor.author | Moragues, Raul | - |
dc.contributor.author | Aparicio, Juan | - |
dc.contributor.author | Esteve, Miriam | - |
dc.contributor.other | Departamentos de la UMH::Estadística, Matemáticas e Informática | es_ES |
dc.date.accessioned | 2025-01-20T18:55:17Z | - |
dc.date.available | 2025-01-20T18:55:17Z | - |
dc.date.created | 2023 | - |
dc.identifier.citation | Mathematics | es_ES |
dc.identifier.issn | 2227-7390 | - |
dc.identifier.uri | https://hdl.handle.net/11000/35050 | - |
dc.description.abstract | Abstract: In this paper, we propose and compare new methodologies for ranking the importance of variables in productive processes via an adaptation of OneClass Support Vector Machines. In particular, we adapt two methodologies inspired by the machine learning literature: one involving the random shuffling of values of a variable and another one using the objective value of the dual formulation of the model. Additionally, we motivate the use of these type of algorithms in the production context and compare their performance via a computational experiment. We observe that the methodology based on shuffling the values of a variable outperforms the methodology based on the dual formulation. We observe that the shuffling-based methodology correctly ranks the variables in 94% of the scenarios with one relevant input and one irrelevant input. Moreover, it correctly ranks each variable in at least 65% of replications of a scenario with three relevant inputs and one irrelevant input. | es_ES |
dc.format | application/pdf | es_ES |
dc.format.extent | 24 | es_ES |
dc.language.iso | eng | es_ES |
dc.publisher | MDPI | es_ES |
dc.relation.ispartofseries | 11 | es_ES |
dc.relation.ispartofseries | 11 | es_ES |
dc.rights | info:eu-repo/semantics/openAccess | es_ES |
dc.rights | Attribution-NonCommercial-NoDerivatives 4.0 Internacional | * |
dc.rights.uri | http://creativecommons.org/licenses/by-nc-nd/4.0/ | * |
dc.subject | data envelopment analysis | es_ES |
dc.subject | feature ranking | es_ES |
dc.subject | model specification | es_ES |
dc.subject | unsupervised machine learning | es_ES |
dc.subject | technical efficiency | es_ES |
dc.subject | overfitting | es_ES |
dc.subject.other | CDU::5 - Ciencias puras y naturales::51 - Matemáticas | es_ES |
dc.title | Ranking the Importance of Variables in a Nonparametric Frontier Analysis Using Unsupervised Machine Learning Techniques | es_ES |
dc.type | info:eu-repo/semantics/article | es_ES |
dc.relation.publisherversion | https://doi.org/10.3390/math11112590 | es_ES |
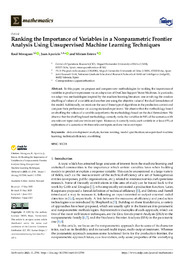
Ver/Abrir:
mathematics-11-02590-v2-1.pdf
481,38 kB
Adobe PDF
Compartir:
La licencia se describe como: Atribución-NonComercial-NoDerivada 4.0 Internacional.