Por favor, use este identificador para citar o enlazar este ítem:
https://hdl.handle.net/11000/34722
Registro completo de metadatos
Campo DC | Valor | Lengua/Idioma |
---|---|---|
dc.contributor.author | Aznar-Tortonda, Vanessa | - |
dc.contributor.author | Palazón-Bru, Antonio | - |
dc.contributor.author | Folgado de la Rosa, David Manuel | - |
dc.contributor.author | Espínola-Morel, Virginia | - |
dc.contributor.author | Pérez-Pérez, Bierca Fermina | - |
dc.contributor.author | León Ruiz, Ana Belén | - |
dc.contributor.author | Gil-Guillén, Vicente F | - |
dc.contributor.other | Departamentos de la UMH::Medicina Clínica | es_ES |
dc.date.accessioned | 2025-01-16T19:30:16Z | - |
dc.date.available | 2025-01-16T19:30:16Z | - |
dc.date.created | 2020-01 | - |
dc.identifier.citation | The British journal of general practice : the journal of the Royal College of General Practitioners. 2019 Dec 26;70(690):e29-e35 | es_ES |
dc.identifier.issn | 1478-5242 | - |
dc.identifier.issn | 0960-1643 | - |
dc.identifier.uri | https://hdl.handle.net/11000/34722 | - |
dc.description.abstract | Background.The main instruments used to assess frailty are the Fried frailty phenotype and the Fatigue, Resistance, Ambulation, Illnesses, and Loss of Weight (FRAIL) scale. Both instruments contain items that must be obtained in a personal interview and cannot be used with an electronic medical record only. Aim. To develop and internally validate a prediction model, based on a points system and integrated in an application (app) for Android, to predict frailty using only variables taken from a patient’s clinical history. Design and setting. A cross-sectional observational study undertaken across the Valencian Community, Spain. Method. A sample of 621 older patients was analysed from January 2017 to May 2018. The main variable was frailty measured using the FRAIL scale. Candidate predictors were: sex, age, comorbidities, or clinical situations that could affect daily life, polypharmacy, and hospital admission in the last year. A total of 3472 logistic regression models were estimated. The model with the largest area under the receiver operating characteristic curve (AUC) was selected and adapted to the points system. This system was validated by bootstrapping, determining discrimination (AUC), and calibration (smooth calibration). Results. A total of 126 (20.3%) older people were identified as being frail. The points system had an AUC of 0.78 and included as predictors: sex, age, polypharmacy, hospital admission in the last year, and diabetes. Calibration was satisfactory. Conclusion. A points system was developed to predict frailty in older people using parameters that are easy to obtain and recorded in the clinical history. Future research should be carried out to externally validate the constructed model. | es_ES |
dc.format | application/pdf | es_ES |
dc.format.extent | 7 | es_ES |
dc.language.iso | eng | es_ES |
dc.publisher | British Journal of General Practice | es_ES |
dc.rights | info:eu-repo/semantics/openAccess | es_ES |
dc.rights | Attribution-NonCommercial-NoDerivatives 4.0 Internacional | * |
dc.rights.uri | http://creativecommons.org/licenses/by-nc-nd/4.0/ | * |
dc.subject | frail elderly | es_ES |
dc.subject | frailty | es_ES |
dc.subject | general practice | es_ES |
dc.subject | mobile applications | es_ES |
dc.subject | statistical models | es_ES |
dc.title | Detection of frailty in older patients using a mobile app: cross-sectional observational study in primary care | es_ES |
dc.type | info:eu-repo/semantics/article | es_ES |
dc.relation.publisherversion | 10.3399/bjgp19X706577 | es_ES |
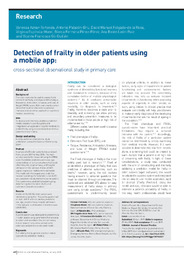
Ver/Abrir:
Detection of frailty in older patients using a mobile app cross-sectional observational study in primary care.pdf
129,94 kB
Adobe PDF
Compartir:
La licencia se describe como: Atribución-NonComercial-NoDerivada 4.0 Internacional.