Please use this identifier to cite or link to this item:
https://hdl.handle.net/11000/34140
Full metadata record
DC Field | Value | Language |
---|---|---|
dc.contributor.author | Cordero, Alberto | - |
dc.contributor.author | Bertomeu-González, Vicente | - |
dc.contributor.author | Segura, José V | - |
dc.contributor.author | Morales-Socuéllamos, Javier | - |
dc.contributor.author | Álvarez-Álvarez, Belén | - |
dc.contributor.author | Escribano, David | - |
dc.contributor.author | Rodríguez-Manero, Moisés | - |
dc.contributor.author | Cid-Alvarez, Belén | - |
dc.contributor.author | García-Acuna, José M. | - |
dc.contributor.author | González Juanatey, José Ramón | - |
dc.contributor.author | Martínez Mayoral, Mª Asunción | - |
dc.contributor.other | Departamentos de la UMH::Estadística, Matemáticas e Informática | es_ES |
dc.date.accessioned | 2024-12-13T09:53:28Z | - |
dc.date.available | 2024-12-13T09:53:28Z | - |
dc.date.created | 2024-08 | - |
dc.identifier.citation | Medicina Clínica, Volume 163, Issue 4, 30 August 2024, Pages 167-174 | es_ES |
dc.identifier.issn | 1578-8989 | - |
dc.identifier.issn | 0025-7753 | - |
dc.identifier.uri | https://hdl.handle.net/11000/34140 | - |
dc.description.abstract | Introducción: La cardiopatía isquémica es la primera causa de insuficiencia cardíaca (IC), y se necesitan herramientas para identificar a los pacientes con mayor probabilidad de desarrollar IC tras un síndrome coronario agudo (SCA). La inteligencia artificial (IA) ha demostrado ser útil para identificar variables relacionadas con el desarrollo de complicaciones cardiovasculares. Métodos: Incluimos todos los pacientes consecutivos dados de alta tras SCA en dos centros espanoles ˜ entre 2006 y 2017. Se recopilaron datos clínicos y se realizó un seguimiento de los pacientes durante una mediana de 53 meses. Los modelos de árboles de decisión fueron creados por el algoritmo de partición recursivo basado en modelos. Resultados: La cohorte fue de 7.097 pacientes, con una mediana de seguimiento de 53 meses (rango intercuartílico: 18-77). La tasa de reingreso por IC fue del 13,6% (964 pacientes). Se identificaron ocho variables relevantes para predecir el tiempo de hospitalización por IC: IC en la hospitalización índice, diabetes, fibrilación auricular, tasa de filtración glomerular, edad, índice de Charlson, hemoglobina y fracción de eyección del ventrículo izquierdo. El modelo de árbol de decisiones proporcionó 15 patrones de riesgo clínico con tasas de reingreso por IC estadísticamente diferentes. Conclusiones: El modelo de árbol de decisión, obtenido por IA, identificó ocho variables principales capaces de predecir IC y generó 15 patrones clínicos diferenciados con respecto a la probabilidad de ser hospitalizado por IC. Se creó una aplicación electrónica gratuita | es_ES |
dc.description.abstract | Background: Coronary heart disease is the leading cause of heart failure (HF), and tools are needed to identify patients with a higher probability of developing HF after an acute coronary syndrome (ACS). Artificial intelligence (AI) has proven to be useful in identifying variables related to the development of cardiovascular complications. Methods: We included all consecutive patients discharged after ACS in two Spanish centers between 2006 and 2017. Clinical data were collected and patients were followed up for a median of 53 months. Decision tree models were created by the model-based recursive partitioning algorithm. Results: The cohort consisted of 7,097 patients with a median follow-up of 53 months (interquartile range: 18-77). The readmission rate for HF was 13.6% (964 patients). Eight relevant variables were identified to predict HF hospitalization time: HF at index hospitalization, diabetes, atrial fibrillation, glomerular filtration rate, age, Charlson index, hemoglobin, and left ventricular ejection fraction. The decision tree model provided 15 clinical risk patterns with significantly different HF readmission rates. Conclusions: The decision tree model, obtained by AI, identified 8 leading variables capable of predicting HF and generated 15 differentiated clinical patterns with respect to the probability of being hospitalized for HF. An electronic application was created and made available for free. | es_ES |
dc.format | application/pdf | es_ES |
dc.format.extent | 8 | es_ES |
dc.language.iso | spa | es_ES |
dc.publisher | Elsevier | es_ES |
dc.rights | info:eu-repo/semantics/closedAccess | es_ES |
dc.rights.uri | http://creativecommons.org/licenses/by-nc-nd/4.0/ | * |
dc.subject | Acute coronary syndrome | es_ES |
dc.subject | Heart failure | es_ES |
dc.subject | Artificial intelligence | es_ES |
dc.subject | Decision tree model | es_ES |
dc.subject | Machine learning | es_ES |
dc.subject | Síndrome coronario agudo | es_ES |
dc.subject | Insuficiencia cardiaca | es_ES |
dc.subject | Inteligencia artificial | es_ES |
dc.subject | Modelo de árbol de decisión | es_ES |
dc.subject | Aprendizaje automático | es_ES |
dc.subject.other | CDU::5 - Ciencias puras y naturales::50 - Generalidades sobre las ciencias puras | es_ES |
dc.title | Árboles de clasificación obtenidos mediante inteligencia artificial para la predicción de insuficiencia cardiaca tras el síndrome coronario agudo | es_ES |
dc.title.alternative | Classification tree obtained by artificial intelligence for the prediction of heart failure after acute coronary syndromes | es_ES |
dc.type | info:eu-repo/semantics/article | es_ES |
dc.relation.publisherversion | https://doi.org/10.1016/j.medcli.2024.01.040 | es_ES |
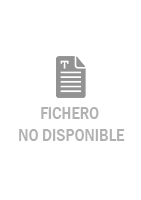
medicina clinica 2024.pdf
1,09 MB
Adobe PDF
Share: