Por favor, use este identificador para citar o enlazar este ítem:
https://hdl.handle.net/11000/31226
Registro completo de metadatos
Campo DC | Valor | Lengua/Idioma |
---|---|---|
dc.contributor.author | López García, Miguel | - |
dc.contributor.author | VALERO, SERGIO | - |
dc.contributor.author | Sans Treserras, Carlos | - |
dc.contributor.author | Senabre, Carolina | - |
dc.date.accessioned | 2024-02-07T13:36:10Z | - |
dc.date.available | 2024-02-07T13:36:10Z | - |
dc.date.created | 2020-12-26 | - |
dc.identifier.citation | Energies 2021, 14, 95 | es_ES |
dc.identifier.issn | 1966-1073 | - |
dc.identifier.uri | https://hdl.handle.net/11000/31226 | - |
dc.description.abstract | This paper introduces a new methodology to include daylight information in short-term load forecasting (STLF) models. The relation between daylight and power consumption is obvious due to the use of electricity in lighting in general. Nevertheless, very few STLF systems include this variable as an input. In addition, an analysis of one of the current STLF models at the Spanish Transmission System Operator (TSO), shows two humps in its error profile, occurring at sunrise and sunset times. The new methodology includes properly treated daylight information in STLF models in order to reduce the forecasting error during sunrise and sunset, especially when daylight savings time (DST) one-hour time shifts occur. This paper describes the raw information and the linearization method needed. The forecasting model used as the benchmark is currently used at the TSO’s headquarters and it uses both autoregressive (AR) and neural network (NN) components. The method has been designed with data from the Spanish electric system from 2011 to 2017 and tested over 2018 data. The results include a justification to use the proposed linearization over other techniques as well as a thorough analysis of the forecast results yielding an error reduction in sunset hours from 1.56% to 1.38% for the AR model and from 1.37% to 1.30% for the combined forecast. In addition, during the weeks in which DST shifts are implemented, sunset error drops from 2.53% to 2.09%. | es_ES |
dc.format | application/pdf | es_ES |
dc.format.extent | 14 | es_ES |
dc.language.iso | eng | es_ES |
dc.publisher | MDPI | es_ES |
dc.rights | info:eu-repo/semantics/openAccess | es_ES |
dc.rights | Attribution-NonCommercial-NoDerivatives 4.0 Internacional | * |
dc.rights.uri | http://creativecommons.org/licenses/by-nc-nd/4.0/ | * |
dc.subject | daylight | es_ES |
dc.subject | load forecasting | es_ES |
dc.subject | power demand | es_ES |
dc.subject.classification | Ingeniería Mecánica | es_ES |
dc.subject.other | CDU::6 - Ciencias aplicadas::62 - Ingeniería. Tecnología::621 - Ingeniería mecánica en general. Tecnología nuclear. Electrotecnia. Maquinaria | es_ES |
dc.title | Use of Available Daylight to Improve Short-Term Load Forecasting Accuracy | es_ES |
dc.type | info:eu-repo/semantics/article | es_ES |
dc.relation.publisherversion | https://dx.doi.org/10.3390/en14010095 | es_ES |
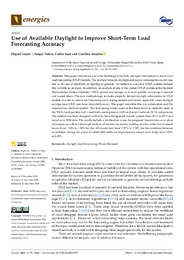
Ver/Abrir:
2021 - Energies - MDPI.pdf
567,02 kB
Adobe PDF
Compartir:
La licencia se describe como: Atribución-NonComercial-NoDerivada 4.0 Internacional.