Please use this identifier to cite or link to this item:
https://hdl.handle.net/11000/30981
Full metadata record
DC Field | Value | Language |
---|---|---|
dc.contributor.author | López, Miguel | - |
dc.contributor.author | VALERO, SERGIO | - |
dc.contributor.author | Senabre, Carolina | - |
dc.contributor.author | Gabaldon, Antonio | - |
dc.contributor.other | Departamentos de la UMH::Ingeniería Mecánica y Energía | es_ES |
dc.date.accessioned | 2024-02-02T16:35:55Z | - |
dc.date.available | 2024-02-02T16:35:55Z | - |
dc.date.created | 2018-06-27 | - |
dc.identifier.issn | 2165-4093 | - |
dc.identifier.uri | https://hdl.handle.net/11000/30981 | - |
dc.description.abstract | In the past decade, many techniques ranging from statistical methods to complex artificial intelligence systems have been proposed by implementing their application to an electric system and highlighting its performance; usually providing a measure of accuracy like RMSE over a definite period. However, there is little research in which a fair comparison among methods is demonstrated, and it is difficult to determine which method would be better suited to a particular electric system or data set. This paper analysis one of the forecasting models running at the National Transport Operator of the Spanish system (REE), which is based on both autoregressive and neural network techniques. The results of this paper help to determine under which circumstances each of the models shows a better performance, which periods are more accurately forecasted by each model and provide valid criteria to choose one or the other depending on the characteristics of the application. | es_ES |
dc.format | application/pdf | es_ES |
dc.format.extent | 5 | es_ES |
dc.language.iso | eng | es_ES |
dc.publisher | Institute of Electrical and Electronics Engineers | es_ES |
dc.relation.ispartof | 2018 15th International Conference on the European Energy Market (EEM) | es_ES |
dc.rights | info:eu-repo/semantics/openAccess | es_ES |
dc.rights | Attribution-NonCommercial-NoDerivatives 4.0 Internacional | * |
dc.rights.uri | http://creativecommons.org/licenses/by-nc-nd/4.0/ | * |
dc.subject | autoregressive processes | es_ES |
dc.subject | demand forecasting | es_ES |
dc.subject | neural networks | es_ES |
dc.subject | power demand | es_ES |
dc.subject.classification | Ingeniería eléctrica | es_ES |
dc.subject.other | CDU::6 - Ciencias aplicadas::62 - Ingeniería. Tecnología | es_ES |
dc.title | Comparison of Short-Term Load Forecasting Performance by Neural Network and Autoregressive Based Models | es_ES |
dc.type | info:eu-repo/semantics/other | es_ES |
dc.relation.publisherversion | https://doi.org/10.1109/EEM.2018.8469797 | es_ES |
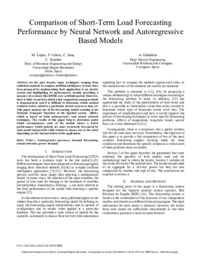
View/Open:
Paper 1 congreso -- Comparison_of_Short-Term_Load_Forecasting_Performance_by_Neural_Network_and_Autoregressive_Based_Models.pdf
1,54 MB
Adobe PDF
Share: