Please use this identifier to cite or link to this item:
https://hdl.handle.net/11000/30796
Full metadata record
DC Field | Value | Language |
---|---|---|
dc.contributor.author | Ribeiro Amaral, André Victor | - |
dc.contributor.author | González, Jonatan A. | - |
dc.contributor.author | Moraga, Paula | - |
dc.contributor.other | Departamentos de la UMH::Estadística, Matemáticas e Informática | es_ES |
dc.date.accessioned | 2024-01-26T22:28:33Z | - |
dc.date.available | 2024-01-26T22:28:33Z | - |
dc.date.created | 2022-12-13 | - |
dc.identifier.citation | Stochastic Environmental Research and Risk Assessment (2023) 37:1519–1533 | es_ES |
dc.identifier.issn | 1436-3259 | - |
dc.identifier.issn | 1436-3240 | - |
dc.identifier.uri | https://hdl.handle.net/11000/30796 | - |
dc.description.abstract | Infectious disease modeling plays an important role in understanding disease spreading dynamics and can be used for prevention and control. The well-known SIR (Susceptible, Infected, and Recovered) compartment model and spatial and spatio-temporal statistical models are common choices for studying problems of this kind. This paper proposes a spatio-temporal modeling framework to characterize infectious disease dynamics by integrating the SIR compartment and log-Gaussian Cox process (LGCP) models. The method’s performance is assessed via simulation using a combination of real and synthetic data for a region in São Paulo, Brazil. We also apply our modeling approach to analyze COVID-19 dynamics in Cali, Colombia. The results show that our modified LGCP model, which takes advantage of information obtained from the previous SIR modeling step, leads to a better forecasting performance than equivalent models that do not do that. Finally, the proposed method also allows the incorporation of age-stratified contact information, which provides valuable decision-making insights. | es_ES |
dc.format | application/pdf | es_ES |
dc.format.extent | 15 | es_ES |
dc.language.iso | eng | es_ES |
dc.publisher | Springer | es_ES |
dc.rights | info:eu-repo/semantics/closedAccess | es_ES |
dc.rights | Attribution-NonCommercial-NoDerivatives 4.0 Internacional | * |
dc.rights.uri | http://creativecommons.org/licenses/by-nc-nd/4.0/ | * |
dc.subject | Compartment SIR model | es_ES |
dc.subject | Infectious diseases | es_ES |
dc.subject | Log-Gaussian Cox process | es_ES |
dc.subject | Spatial point process | es_ES |
dc.subject | Spatio-temporal modeling | es_ES |
dc.subject.classification | Estadística e investigación operativa | es_ES |
dc.subject.other | CDU::3 - Ciencias sociales::31 - Demografía. Sociología. Estadística::311 - Estadística | es_ES |
dc.title | Spatio-temporal modeling of infectious diseases by integrating compartment and point process models | es_ES |
dc.type | info:eu-repo/semantics/article | es_ES |
dc.relation.publisherversion | https://doi.org/10.1007/s00477-022-02354-4 | es_ES |
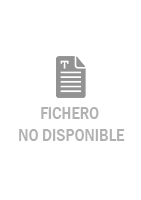
2022 Ribeiro et al - Spatio-temporal modeling of infectious diseases by integrating.pdf
2,14 MB
Adobe PDF
Share: